The Complete Guide to Revenue Growth Management in Consumer Packaged Goods
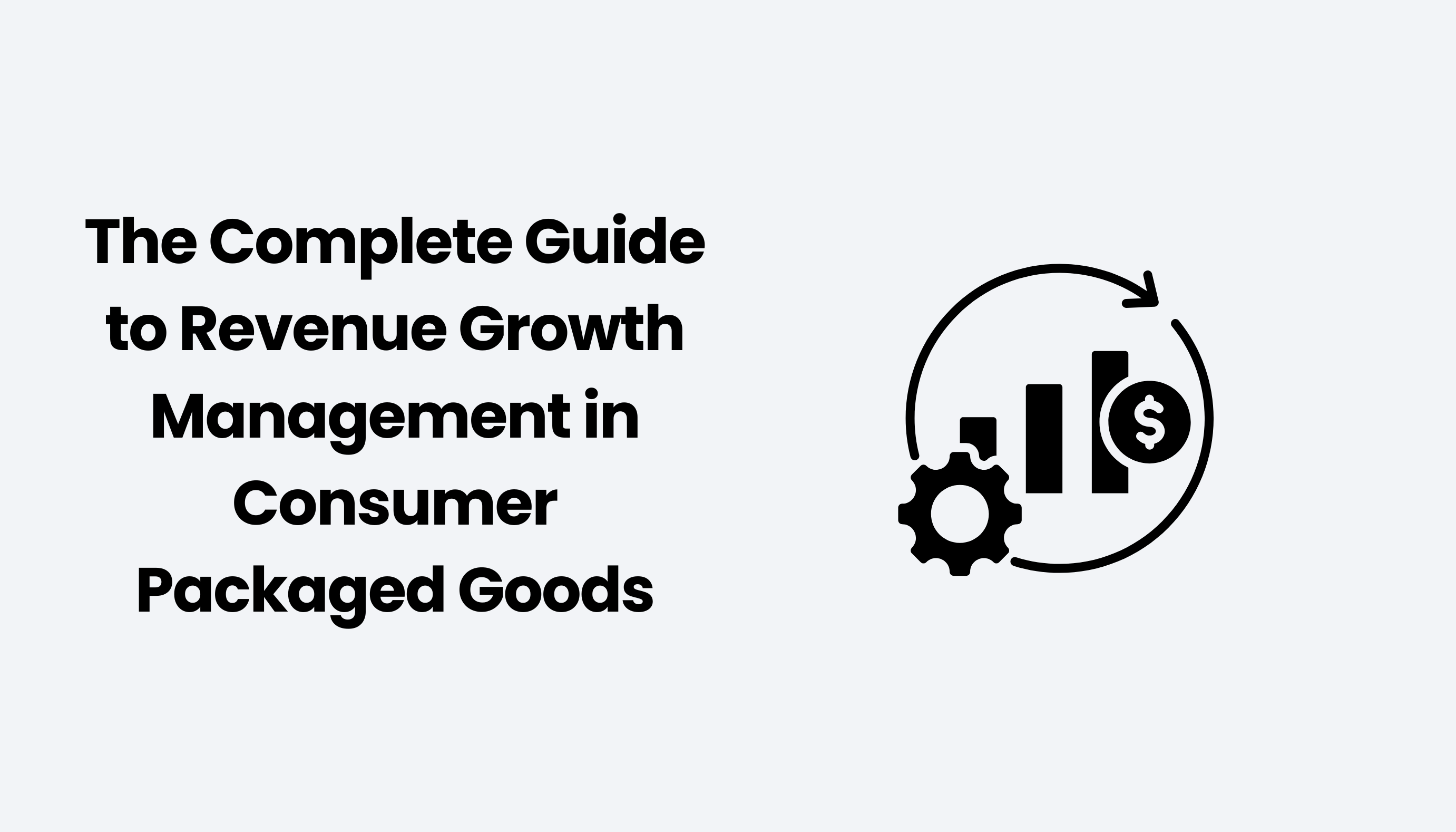
Revenue Growth Management (RGM) has become a critical function for Consumer Packaged Goods (CPG) companies striving to maintain profitability in an increasingly competitive market. Traditional pricing and promotional tactics are no longer sufficient as consumer behavior shifts, digital commerce expands, and data-driven decision-making becomes essential. RGM provides a structured approach to optimizing pricing, promotions, trade spend, and brand positioning to maximize revenue while maintaining long-term brand equity.
This guide explores the core pillars of RGM, key market factors influencing revenue strategies, common pitfalls that hinder growth, and how AI and analytics are reshaping the field.
Table of Contents:
- What is Revenue Growth Management (RGM) in CPG?
- How RGM Has Evolved from Traditional Trade Promotion Strategies?
- Why RGM is Essential for Modern CPG Businesses?
- The Core Pillars of Revenue Growth Management in CPG
- The Key Factors Impacting RGM in CPG
- Common Pitfalls of Traditional RGM and How to Overcome Them
- How AI and Data Analytics is Changing RGM?
- Revenue Growth Management Use Cases in the CPG Industry
- The Future of Revenue Growth Management in CPG
- Getting Started with an Effective RGM Strategy
Jump to a section that interests you, or keep reading.
What is Revenue Growth Management (RGM) in CPG?
Revenue Growth Management (RGM) in the Consumer Packaged Goods (CPG) industry refers to the strategic use of pricing, promotions, trade spend, and assortment optimization to maximize revenue and profitability. It involves data-driven decision-making to align product pricing and promotional strategies with consumer demand, competitive dynamics, and retailer expectations. Unlike traditional sales-driven approaches, RGM integrates analytics, AI, and real-time market insights to refine pricing structures, enhance trade efficiency, and ensure sustainable growth.
How RGM Has Evolved from Traditional Trade Promotion Strategies?
Historically, CPG companies relied heavily on trade promotions and discounts to drive volume. However, these methods often led to margin erosion, inefficient spending, and a lack of transparency in ROI. Traditional trade promotion strategies were reactive, dependent on historical data, and lacked the flexibility to adapt to changing market conditions.
The shift towards RGM was driven by the need for greater profitability, precision, and predictability in revenue planning. Companies started incorporating price pack architecture, demand forecasting, and AI-driven analytics to optimize pricing decisions. Advanced trade promotion optimization (TPO) tools replaced broad, high-discount campaigns with targeted, data-backed promotions that aligned with consumer behavior. Additionally, real-time analytics allowed businesses to measure promotional effectiveness and adjust strategies dynamically, improving both short-term sales impact and long-term brand value.
Why RGM is Essential for Modern CPG Businesses?
RGM is critical in today’s competitive and margin-constrained CPG environment. Several factors make it indispensable:
- Changing Consumer Behavior: Shoppers are more price-sensitive and selective, requiring brands to refine pricing strategies to maintain loyalty while preserving margins.
- Rising Competition: The proliferation of private labels and direct-to-consumer (DTC) brands has intensified pricing pressure, necessitating smarter revenue management to stay competitive.
- Omnichannel Complexity: Consumers engage across multiple channels, making it essential to align pricing and promotions across e-commerce, brick-and-mortar, and direct sales.
- AI and Big Data Advancements: Modern RGM strategies leverage AI-driven pricing models, demand forecasting, and real-time analytics to enhance decision-making.
- Retailer Negotiations: Trade spend must be allocated strategically to maximize retailer relationships while ensuring profitability.
The Core Pillars of Revenue Growth Management in CPG
Pricing Strategy
Consumer perception of price directly influences purchasing behavior and brand loyalty. A poorly positioned price can either erode margins or push consumers toward competitors. Understanding how consumers interpret price relative to value ensures that pricing strategies align with market expectations.
Analyzing how price changes impact demand is essential for optimizing revenue. Price elasticity studies help brands determine the optimal price points that maximize sales volume without sacrificing margins. High elasticity indicates that demand is highly sensitive to price changes, requiring a cautious approach to discounting and pricing adjustments.
AI-driven dynamic pricing models allow brands to adjust prices in real time based on market conditions, competitor pricing, and consumer demand patterns. These models enable more precise revenue management by ensuring that pricing remains competitive while maintaining profitability.
Optimizing pack sizes and price points for different consumer segments is a fundamental part of revenue growth management. Offering various pack sizes at different price tiers allows brands to cater to budget-conscious consumers while maximizing per-unit profitability. Well-structured price pack architecture balances accessibility and margin optimization across different channels.
Trade Promotion Optimization (TPO)
Trade promotions are critical in influencing consumer purchasing decisions and retailer engagement. Effective promotions can boost short-term sales and improve shelf visibility. However, without a strategic approach, excessive discounting can reduce profitability without delivering long-term brand benefits.
Many CPG brands struggle with ineffective promotions due to poor targeting, lack of data-driven planning, and over-reliance on discounting. Blanket promotions often fail to generate incremental sales and instead lead to subsidized purchases that would have occurred at full price.
Analyzing historical promotional performance helps brands predict the impact of future campaigns. Response modeling leverages AI and machine learning to assess which types of promotions drive true volume growth and which ones merely shift existing demand.
Strategic allocation of promotional budgets ensures that spending is directed toward initiatives with the highest return on investment. Optimizing the mix between discounts, in-store activations, and digital promotions prevents wasteful spending while maximizing revenue impact.
Trade Spend Management
Trade spend is one of the largest budgetary components for CPG companies, directly influencing profitability. Poorly managed trade investments can lead to revenue leakage, while a well-structured trade spend strategy ensures that funds drive incremental sales and strengthen retailer relationships.
Data-driven trade spend allocation prioritizes investments that yield the highest returns. AI-powered analytics enable brands to measure the effectiveness of past trade spend and reallocate budgets accordingly to maximize efficiency.
Effective negotiation with retailers ensures that promotional investments align with brand objectives rather than solely benefiting the retailer. Structured trade agreements should balance promotional support with margin protection to avoid unnecessary profit dilution.
Continuous tracking of trade spend performance helps identify underperforming investments and refine future spending. Advanced analytics provide insights into how trade spend contributes to volume growth, margin improvements, and overall brand performance.
Brand Positioning & Consumer Insights
Revenue Growth Management plays a crucial role in reinforcing brand positioning by ensuring that pricing, promotions, and trade investments align with the brand’s perceived value. A well-executed RGM strategy prevents price erosion and maintains premium brand positioning.
Understanding what motivates consumers to choose one product over another is key to refining pricing and promotional strategies. Consumer insights help brands identify trends, assess price sensitivity, and develop targeted marketing approaches.
Assessing competitor pricing, promotions, and market positioning allows brands to make informed decisions that enhance their competitive advantage. Data-driven benchmarking ensures that pricing and promotional strategies remain relevant in a dynamic market.
Consumer expectations and market conditions are constantly evolving. Brands must continuously reassess their positioning, pricing, and promotional strategies to stay competitive while maintaining profitability.
Marketing Mix Optimization
CPG brands operate across multiple sales channels, including e-commerce, retail stores, and direct-to-consumer platforms. A well-balanced budget allocation ensures that resources are distributed based on each channel’s revenue contribution and growth potential.
Tracking campaign performance across digital and traditional channels provides insights into ROI and consumer engagement. Data analytics help refine future marketing investments by identifying the most effective messaging and promotional tactics.
Advanced scenario modeling allows brands to predict how different promotional strategies will impact sales and profitability. By simulating various pricing and promotional approaches, brands can make informed decisions that optimize revenue while managing risks.
The Key Factors Impacting RGM in CPG
Changing Consumer Behavior and Market Trends
Shifts in consumer preferences have significantly altered revenue growth management strategies in the CPG sector. Consumers are more price-sensitive, seeking better value while expecting high-quality products. Private labels have gained market share, putting pressure on branded products to justify their price premiums. Additionally, demand for sustainable, health-conscious, and personalized products has reshaped how companies position their offerings. Shopping habits have also evolved, with digital channels playing a larger role in product discovery and purchase decisions. As a result, traditional volume-driven pricing and promotion strategies must be adapted to meet these new behavioral patterns.
Increased Competition and Margin Pressures
The CPG industry faces intensifying competition from both established brands and emerging players, including niche direct-to-consumer brands and private-label products. Retailers, aiming to maximize margins, push for better pricing and trade terms, making it challenging for brands to maintain profitability. Cost pressures from inflation, supply chain disruptions, and rising raw material expenses further strain margins. Companies must carefully manage pricing, promotions, and trade investments to balance volume growth with profitability. Margin optimization requires data-driven decision-making to ensure price adjustments and trade spend allocations enhance revenue without eroding brand equity.
The Impact of E-Commerce and Direct-to-Consumer (DTC) Models
The shift toward online shopping has transformed traditional revenue growth strategies. E-commerce allows for dynamic pricing, personalized promotions, and direct consumer engagement, but also increases competition and price transparency. The rise of DTC brands has further disrupted traditional distribution models by offering lower prices and a direct relationship with consumers. This has forced legacy brands to refine their pricing strategies across channels to prevent revenue dilution. Managing omnichannel pricing consistency, optimizing digital trade spend, and leveraging data from e-commerce transactions are now essential for maintaining competitiveness and profitability.
The Role of AI and Analytics in Optimizing RGM
AI and advanced analytics have become central to revenue growth management, providing real-time insights into pricing, promotions, and consumer behavior. AI-driven pricing models enable dynamic adjustments based on demand patterns, competitive activity, and market conditions. Machine learning enhances demand forecasting, allowing for more precise inventory planning and reducing stockouts or overstock situations. AI-powered analytics also improve trade promotion optimization by identifying which promotions drive incremental sales rather than subsidizing existing demand. Additionally, AI-driven customer segmentation enables targeted marketing strategies that improve retention and acquisition. The integration of AI ensures that RGM strategies are continuously refined based on real-time data, maximizing both short-term revenue and long-term brand growth.
Common Pitfalls of Traditional RGM and How to Overcome Them
Over-Reliance on Historical Data
Traditional revenue growth management often depends on past sales performance to guide pricing, promotions, and trade spend. This backward-looking approach fails to account for shifting consumer behaviors, emerging competitors, and macroeconomic factors. Relying on outdated models can result in ineffective pricing and promotional strategies. To overcome this, companies must integrate real-time data analytics and AI-driven forecasting to anticipate future demand and optimize revenue strategies accordingly.
Inefficient Trade Promotions
Many CPG companies continue to invest heavily in trade promotions without a clear understanding of their return on investment. Promotional funds are often allocated based on retailer relationships or past spending patterns rather than data-driven insights. This leads to margin erosion and inefficient spending. A structured Trade Promotion Optimization (TPO) framework, leveraging advanced analytics, can help brands measure promotion effectiveness, adjust budgets dynamically, and focus investments on activities that drive true incremental sales.
Lack of Price Elasticity Understanding
Traditional RGM strategies frequently apply broad pricing adjustments across product categories without considering price elasticity at the SKU level. Mispricing—either overpricing or underpricing—can lead to lost sales, lower profitability, or brand devaluation. AI-driven pricing models that analyze price sensitivity in real time allow for dynamic pricing strategies that align with consumer willingness to pay while protecting margins.
Poor Integration Across Channels
Many CPG brands struggle with inconsistent pricing and promotions across online and offline channels, leading to consumer confusion and potential revenue loss. A fragmented approach to e-commerce and brick-and-mortar pricing creates challenges in maintaining brand perception and profitability. A unified omnichannel RGM strategy ensures pricing, trade spend, and promotions are aligned across all touchpoints, improving consumer trust and maximizing revenue opportunities.
Failure to Adapt to E-Commerce and DTC Growth
Traditional RGM often focuses on retail-driven sales models, neglecting the rapid expansion of e-commerce and direct-to-consumer (DTC) channels. Many brands lack a dedicated strategy for digital pricing, personalized promotions, and consumer engagement in these spaces. To remain competitive, companies must develop distinct RGM strategies for online channels, incorporating AI-driven consumer insights and dynamic pricing tailored to digital shoppers.
Siloed Decision-Making
In many organizations, revenue growth management operates in isolation, with pricing, promotions, trade spend, and marketing decisions being made separately. This disconnect leads to inefficiencies and suboptimal revenue outcomes. A cross-functional RGM team, supported by integrated data platforms, enables holistic decision-making that aligns pricing, promotions, and trade investments with broader business objectives.
Inadequate Measurement of RGM Effectiveness
Traditional RGM approaches often lack robust performance tracking, making it difficult to assess the impact of pricing changes, promotions, and trade spend investments. Without clear KPIs, companies risk continuing ineffective strategies. Implementing real-time analytics dashboards and performance measurement frameworks allows brands to track revenue growth drivers, adjust tactics quickly, and improve decision-making accuracy.
How AI and Data Analytics is Changing RGM?
AI-Driven Pricing Strategies
Traditional pricing models rely on historical data and broad market trends, often leading to suboptimal price points that either erode margins or reduce sales volume. AI-driven pricing strategies analyze real-time market conditions, competitive pricing, and consumer purchasing behavior to determine the most profitable price points for different SKUs. Machine learning algorithms continuously refine pricing based on demand elasticity, allowing brands to implement dynamic pricing models that maximize revenue across various channels. AI also identifies price thresholds that consumers perceive as acceptable, preventing unnecessary discounting and maintaining brand equity.
Machine Learning Models for Demand Forecasting
Accurate demand forecasting is critical to ensuring product availability while minimizing excess inventory costs. Traditional forecasting methods struggle to account for seasonality, regional demand fluctuations, and macroeconomic variables. Machine learning models enhance forecasting accuracy by processing vast datasets, identifying patterns, and adjusting projections in real time. AI-driven models incorporate weather patterns, social media sentiment, promotional impact, and competitor activity to refine demand predictions. This allows brands to optimize production planning, inventory management, and distribution strategies, reducing stockouts and overproduction.
Real-Time Data Analytics for Promotions and Trade Spend
Trade promotions represent a significant investment for CPG companies, but measuring their effectiveness remains a challenge. AI-driven analytics track real-time promotional performance, identifying which campaigns drive true incremental sales and which erode margins. AI-powered models can also simulate different promotion scenarios, helping brands allocate trade spend more efficiently. Real-time insights enable rapid adjustments to promotion timing, discount levels, and channel-specific offers, ensuring higher ROI on marketing investments. By integrating data from retail scanners, e-commerce platforms, and loyalty programs, AI enhances visibility into consumer response to promotional efforts.
AI-Powered Customer Segmentation and Personalization
Consumer preferences are constantly evolving, making static segmentation strategies ineffective. AI-powered customer segmentation leverages behavioral, demographic, and transactional data to group consumers into micro-segments based on purchasing habits, price sensitivity, and brand loyalty. This granular segmentation allows brands to tailor promotions, personalized pricing, and marketing messages to specific audience clusters, increasing engagement and conversion rates. AI also enhances direct-to-consumer (DTC) personalization, enabling real-time product recommendations and targeted offers based on individual browsing and purchase history.
Revenue Growth Management Use Cases in the CPG Industry
Campaign Performance Measurement
CPG brands allocate significant budgets to promotions and advertising, but measuring their true impact is often complex. AI-driven analytics track real-time sales lift, customer engagement, and incremental revenue generated by each campaign. By integrating point-of-sale data, digital ad performance, and in-store activation metrics, brands gain a clearer picture of which efforts drive actual growth versus those that simply shift demand.
Consumers interact with brands across multiple touchpoints, from social media to in-store promotions. AI-powered attribution models assign revenue impact to each channel, helping brands optimize budget allocation. These models analyze customer journeys, media spend, and conversion paths, ensuring investment in the most profitable channels.
Demand Forecasting for Different Brands & Packs
Traditional forecasting models often fail to capture demand fluctuations caused by seasonality, market shifts, and competitive activity. AI-driven forecasting enhances accuracy by integrating historical sales data, weather conditions, economic indicators, and social sentiment analysis. This enables CPG brands to anticipate demand surges and adjust supply chains proactively.
Stockouts lead to missed revenue opportunities, while overstock increases holding costs. AI-powered inventory optimization tools analyze sell-through rates, retailer replenishment cycles, and distribution patterns to maintain ideal stock levels. Real-time demand signals ensure just-in-time replenishment, reducing lost sales and minimizing waste.
Optimizing Promotions and Pricing
Different consumer segments respond to pricing and bundling strategies in unique ways. AI models assess price sensitivity, purchase frequency, and competitive positioning to create tailored promotions that maximize both volume and margin. Advanced algorithms test different bundle configurations to determine the most effective combinations for various demographics.
Pricing decisions must balance consumer affordability with profitability. AI analyzes elasticity curves across product categories and regions, enabling brands to adjust pricing dynamically without eroding perceived value. Data-driven insights prevent excessive discounting and ensure price changes align with market demand and competitive benchmarks.
Customer Analytics for Retention & Acquisition
Retaining customers is more cost-effective than acquiring new ones. AI-powered models predict churn risk by analyzing past purchase behavior, engagement trends, and external factors like competitor promotions. Brands can then proactively engage at-risk customers with targeted offers and loyalty incentives to extend customer lifetime value.
Personalization increases conversion rates by delivering relevant messaging and offers. AI segments customers based on purchase patterns, demographics, and online behavior, allowing brands to automate tailored promotions, recommend products, and optimize email and ad targeting strategies. Predictive analytics enhance direct-to-consumer (DTC) initiatives, ensuring marketing efforts resonate with specific audience segments.
The Future of Revenue Growth Management in CPG
Emerging Trends Shaping RGM
Sustainability-driven pricing strategies are becoming a priority as consumers increasingly seek environmentally responsible products. Brands are incorporating sustainable packaging, ethically sourced ingredients, and carbon-neutral production into their pricing models. AI is being used to assess consumer willingness to pay for sustainability, ensuring that green pricing aligns with demand while maintaining profitability.
Direct-to-consumer (DTC) models are expanding as brands move away from a heavy reliance on retail partners. This shift allows companies to have greater control over pricing, promotions, and customer data. AI-driven personalization is enhancing DTC strategies, helping brands refine subscription models, dynamic bundling, and targeted discounting to improve retention and lifetime value. E-commerce growth continues to reshape revenue management, requiring brands to optimize pricing strategies to remain competitive.
Real-time AI-powered pricing adjustments are replacing traditional static pricing models. Machine learning continuously refines pricing based on historical sales data, competitor movements, and regional purchasing trends. This enables companies to respond to demand fluctuations and economic conditions, optimizing prices without sacrificing margins. Automated adjustments help brands remain agile in retail environments where seasonal demand and promotions shift frequently.
Personalized promotions are gaining traction as mass-market discounting becomes less effective. Brands are leveraging AI-driven segmentation to create targeted offers based on loyalty programs, online behavior, and purchase history. This ensures that promotions reach the right consumers at the right time, improving engagement while reducing unnecessary markdowns.
The Shift from Tactical RGM to Long-Term Strategic Growth
RGM has traditionally focused on short-term margin optimization through pricing adjustments, promotions, and trade spend allocation. The focus is now shifting toward sustained revenue growth by integrating brand equity, cross-functional collaboration, and predictive analytics. Companies are moving away from reactive pricing and promotions, instead developing a more structured revenue management framework that aligns with brand positioning, changing consumer expectations, and omnichannel expansion.
How CPG Brands Can Prepare for Future RGM Challenges
To stay competitive, brands must invest in AI-driven analytics, enhance direct consumer engagement, and refine data-driven pricing models. Synchronizing RGM efforts across retail, e-commerce, and DTC channels is essential for long-term success. Predictive modeling helps brands anticipate market shifts, ensuring agility in responding to pricing pressures and economic volatility. Companies that adopt scalable AI tools, improve data governance, and foster collaboration across sales, marketing, and finance will be better positioned to manage revenue growth effectively in an evolving market.
Getting Started with an Effective RGM Strategy
Assessing Your Current Revenue Growth Framework
A structured evaluation of the existing revenue growth framework is the first step in establishing an optimized RGM strategy. This involves analyzing historical pricing decisions, trade promotion effectiveness, and trade spend allocation to identify inefficiencies. Understanding price elasticity, margin structures, and consumer purchasing patterns is critical to uncover revenue leakage and missed opportunities. A data-driven approach ensures that brands can diagnose gaps in their strategy and establish a foundation for improvement.
Choosing the Right Tools and Analytics Platforms
Selecting appropriate technology and analytics platforms is essential for executing a scalable RGM strategy. AI-powered pricing tools enable real-time price adjustments based on demand fluctuations, competitive pricing, and consumer behavior. Trade promotion optimization (TPO) software helps in evaluating past promotions and predicting future performance, ensuring marketing budgets are allocated effectively. Advanced trade spend management systems provide granular insights into retailer negotiations, deduction management, and ROI tracking. Integrated analytics platforms allow brands to centralize data from multiple channels, facilitating better decision-making across pricing, promotions, and consumer insights.
Building a Cross-Functional RGM Team
RGM success depends on collaboration across multiple departments, including sales, finance, marketing, and supply chain. A cross-functional team ensures alignment between pricing strategy, promotional planning, brand positioning, and inventory management. Finance teams provide profitability insights, while marketing teams assess promotional effectiveness and consumer engagement. Sales teams contribute retail execution knowledge, and supply chain teams ensure pricing strategies align with production and distribution constraints. This integrated approach minimizes silos, improving efficiency in revenue growth initiatives.
Setting KPIs for Measuring Success
Defining key performance indicators (KPIs) ensures that RGM efforts remain focused and measurable. Core KPIs include revenue per unit, price realization, trade spend ROI, promotion lift, margin improvement, and customer acquisition costs. AI-driven analytics allow brands to track these metrics in real time, identifying trends and adjusting strategies accordingly. Performance benchmarks should be reviewed regularly to refine pricing structures, promotional tactics, and trade spend allocations. By continuously measuring success, companies can maintain a dynamic and responsive RGM strategy that adapts to changing market conditions and consumer behavior.
Conclusion
A well-executed RGM strategy is essential for CPG brands to sustain profitability and adapt to the complexities of modern retail. Companies that embrace AI-driven pricing, demand forecasting, and advanced trade spend management will gain a competitive edge by making smarter, data-backed decisions. At the same time, understanding shifting consumer preferences and optimizing marketing investments will be crucial for long-term success.
As RGM moves from a tactical function to a long-term strategic growth driver, businesses must continuously refine their approach. By integrating advanced analytics, fostering cross-functional collaboration, and maintaining a focus on consumer value, brands can build a sustainable revenue growth framework that not only maximizes short-term gains but also ensures long-term resilience in an evolving marketplace.