How AI is Reshaping the Future of Rebate Management?
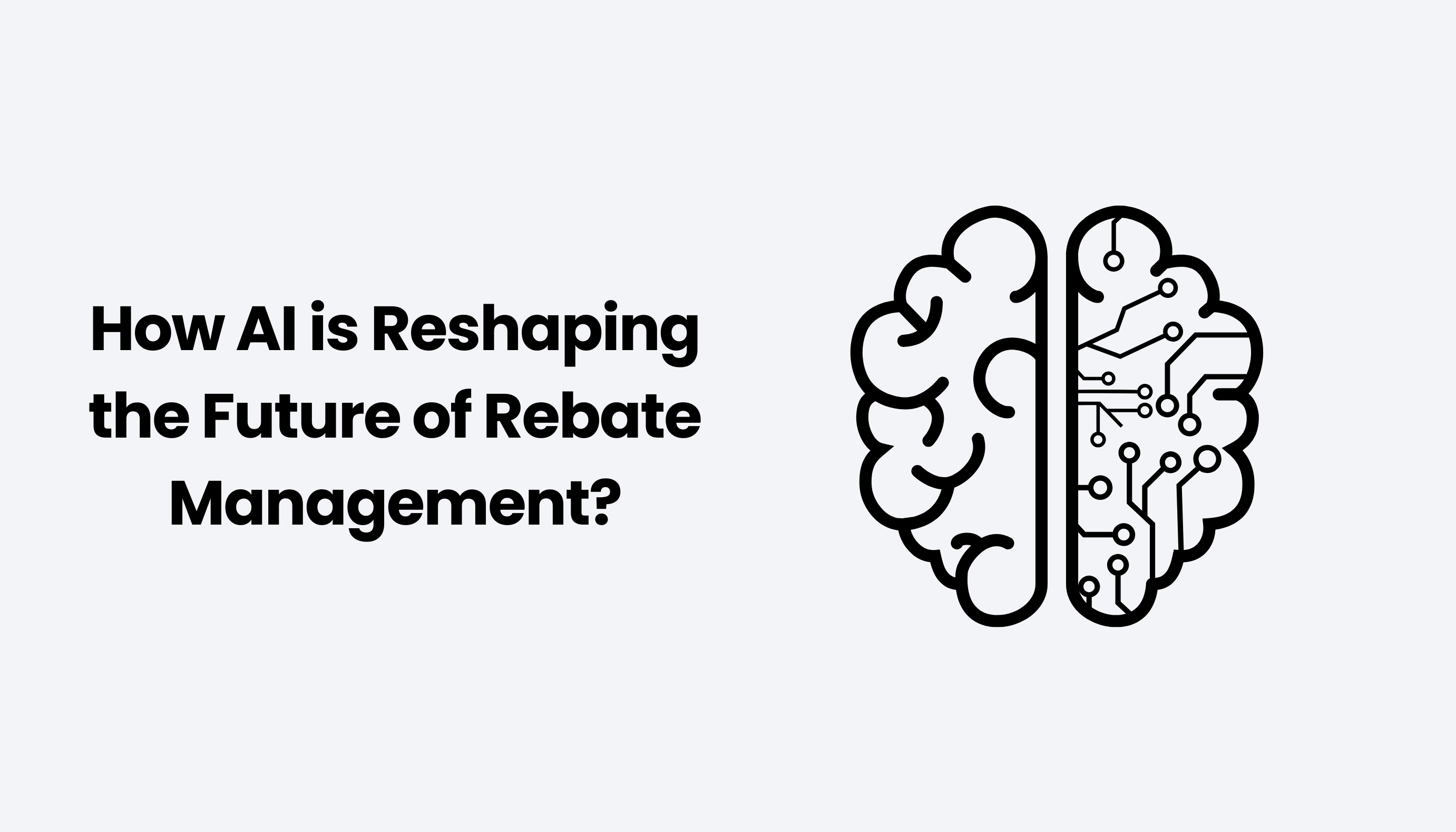
Artificial Intelligence (AI) is reshaping rebate management by introducing an adaptive intelligence layer that transforms how businesses handle rebates. Unlike traditional systems, AI processes historical and real-time data to deliver predictive analytics, scenario modeling, and actionable recommendations.
This guide will help you understand how AI is transforming rebate management to improve operational efficiency.
Table of Contents:
- The Evolution of Rebate Management
- 6 Key AI Capabilities in Rebate Management
- 5 Benefits of AI Tools for Rebate Management
- Addressing 4 Common Challenges with AI in Rebate Management
- 4 Real-World Applications of AI in Rebate Management
- 5 Steps to Implement AI in Your Rebate Strategy
Jump to a section that interests you, or keep reading.
The Evolution of Rebate Management
Historically, rebate management relied heavily on manual workflows, such as spreadsheets, email communications, and basic reporting tools. These methods, though familiar, were highly inefficient and prone to errors. Data silos formed as rebate data was stored across disparate systems or departments, which led to fragmentation that disrupted collaboration and visibility. Manual processes introduced human error, with mistakes in data entry, calculations, or reporting leading to rebate payouts and forecast inaccuracies. These inefficiencies resulted in delays in issuing rebates, which frustrated customers and trading partners. Additionally, businesses struggled to extract meaningful insights from static data, which made it difficult to optimize rebate programs.
The introduction of rebate management software marked a shift away from entirely manual processes. Early digital tools automated some repetitive tasks, such as rebate calculations, reporting, and basic program tracking. This helped businesses to reduce reliance on spreadsheets and manual workflows and improve operational efficiency to an extent. However, these early systems came with some limitations. They lacked real-time data visibility, meaning businesses were often analyzing rebate performance long after critical opportunities had passed. These tools could not provide the advanced insights required for strategic decision-making, such as predicting rebate performance trends or identifying areas for improvement. As rebate programs grew in complexity and data volumes increased, the need for more intelligent and adaptive solutions became evident.
The advent of AI (Artificial Intelligence) and machine learning has fundamentally transformed rebate management by introducing advanced predictive, analytical, and real-time capabilities. Unlike earlier systems, AI-powered tools can process massive volumes of both historical and real-time data to deliver actionable insights. For example, AI enables predictive analytics that forecast rebate performance, customer behavior, and market trends, which help businesses refine rebate structures for maximum profitability. AI also facilitates real-time adjustments, helping companies to respond dynamically to changing market conditions or underperforming programs.
Machine learning algorithms further improve rebate management by identifying patterns, detecting anomalies, and optimizing rebate strategies. AI reduces reliance on manual intervention, automating routine tasks such as data entry, calculations, and reporting while minimizing human error. Businesses now benefit from tools like natural language processing (NLP), which simplify complex data queries and rebate agreement extractions, making advanced analysis accessible to non-technical users.
6 Key AI Capabilities in Rebate Management
AI-Driven Analytics
- Predictive Analytics: AI improves rebate management through predictive analytics by analyzing vast amounts of historical and real-time data to forecast customer behavior and rebate performance. By analyzing patterns and trends, businesses can accurately forecast outcomes, which would help them to make data-backed decisions when designing rebate programs. AI also supports scenario planning, which helps companies simulate various outcomes and assess the impact of changes to rebate structures before implementation. This ensures rebate strategies are optimized to align with both market conditions and organizational goals to mitigate risks and maximize profitability.
- Prescriptive Analytics: AI takes analytics a step further with prescriptive analytics by delivering actionable recommendations to address underperforming rebate programs and improve results. By processing large, complex datasets, AI identifies opportunities for program refinement and offers insights into adjustments that drive better outcomes. These targeted recommendations help organizations optimize rebate strategies so that they are competitive and aligned with customer needs.
- Risk Detection and Anomaly Identification: AI’s advanced algorithms are invaluable for detecting anomalies, errors, and compliance issues within rebate programs. By scanning vast data volumes, AI tools can pinpoint discrepancies in rebate calculations, uncover inaccuracies, and flag inconsistencies that might otherwise go unnoticed. For instance, anomalies in claims, payments, or performance metrics can be identified in real time so that businesses can proactively address risks before they escalate into costly problems. AI also serves as an early warning system for fraud detection to strengthen financial oversight and ensure rebate programs operate transparently and accurately.
Automation of Routine Tasks
AI reduces manual workload by automating repetitive tasks inherent in rebate management. Processes such as data entry, rebate calculations, and reporting, which were once time-consuming and error-prone, are simplified with AI-driven tools. By automating these functions, businesses not only improve accuracy but also minimize the risk of human error that can arise from manual workflows.
This automation improves operational efficiency by helping rebate teams shift their focus from administrative tasks to high-value strategic initiatives. For instance, sales and finance teams can dedicate more time to analyzing performance, refining rebate structures, and building stronger trading partner relationships. The result is improved productivity, better resource allocation, and faster rebate processing, which collectively drive better program outcomes.
Real-Time Adjustments and Insights
AI delivers real-time adjustments and insights to help businesses dynamically adapt rebate programs based on changing market conditions. AI ensures that rebate strategies remain aligned with current business goals and market performance by continuously analyzing live data. This agility allows companies to respond swiftly to opportunities, such as adjusting rebate terms when key performance indicators (KPIs) are achieved or when program performance deviates from expectations.
AI facilitates real-time tracking of rebate performance metrics to provide businesses with up-to-the-minute visibility into program success. Dynamic dashboards and automated alerts allow stakeholders to monitor trends, detect underperformance, and take immediate corrective action. For example, if a rebate program begins to lag, AI tools can flag the issue promptly to support teams in refining strategies on the fly. This instant access to actionable insights empowers organizations to capitalize on opportunities, address risks, and maximize the value of their rebate programs with unprecedented speed and precision.
Personalized Rebate Programs with Machine Learning
Machine learning helps businesses create personalized rebate programs by analyzing customer behavior and purchase history to tailor rebate offerings. By processing vast datasets, machine learning algorithms identify purchasing patterns and preferences, ensuring that rebate incentives resonate with specific customer groups. This level of personalization ensures rebates are more relevant to driving higher engagement and redemption rates.
As these systems continue to gather and process more data over time, they fine-tune rebate structures and delivery mechanisms automatically. For instance, machine learning can adjust rebate types, timing, and delivery channels to maximize their impact based on changing customer behaviors and market dynamics. The result is more accurate targeting and greater program efficiency.
This personalization improves customer satisfaction and loyalty by ensuring customers receive rebates that align with their specific needs and expectations. By delivering tailored offers, businesses build stronger relationships, encourage repeat purchases, and ultimately strengthen their connection with their trading partners.
Advanced Forecasting and Scenario Modeling
AI’s advanced forecasting capabilities allow businesses to run “what-if” simulations to test the outcomes of different rebate program strategies before implementation. By analyzing historical and real-time data, these simulations help organizations predict the potential performance of rebate structures under various scenarios to identify the most profitable options.
The ability to conduct scenario modeling supports proactive adjustments to deal terms before they are finalized. For example, AI can analyze projected sales, market conditions, and customer behavior to recommend changes that optimize rebate performance. This ensures that rebate agreements remain agile, adaptable, and aligned with business objectives.
AI-powered forecasting tools also play an important role in financial planning by providing reliable, data-driven predictions about rebate costs and outcomes. Businesses can anticipate future trends, allocate resources, and ensure that rebate programs align with overall financial goals. This forward-looking approach enables companies to mitigate risks, seize opportunities, and plan with confidence.
Natural Language Processing (NLP)
Natural Language Processing (NLP) simplifies the way businesses interact with rebate data by enabling users to perform complex data queries using plain language questions. Instead of relying on technical expertise, team members can ask conversational questions, such as “Which rebate programs underperformed last quarter?” or “What are the current top-performing rebate deals?” NLP instantly generates accurate, real-time insights to make data analysis more accessible across all levels of the organization.
NLP also automates contract extraction and simplifies the analysis of rebate agreements. AI tools powered by NLP can identify and extract key terms, conditions, and obligations from complex documents to eliminate the need for manual reviews. This accelerates processes, reduces errors, and ensures rebate terms are accurately interpreted and implemented.
By providing real-time insights, NLP helps non-technical users make data-driven decisions without relying on data analysts or IT teams. This accessibility creates a more data-driven culture by ensuring that all stakeholders can engage with rebate data, monitor performance, and drive strategic outcomes.
5 Benefits of AI Tools for Rebate Management
- Accurate Insights and Decision-Making: AI-powered rebate platforms deliver accurate, data-driven insights by analyzing vast datasets to identify trends, uncover hidden opportunities, and provide actionable recommendations. These tools allow businesses to pinpoint underperforming programs, optimize rebate structures, and make decisions that improve financial outcomes. Businesses can confidently respond to market shifts by using real-time analytics so that rebate programs remain aligned with business goals.
- Improved Efficiency: AI significantly improves operational efficiency by automating time-consuming processes like rebate calculations, reporting, and data validation. Faster processing reduces administrative workloads so that teams can focus on strategic tasks instead of repetitive, manual activities. With automation, businesses eliminate human errors that often result from manual workflows to ensure rebate programs are managed accurately.
- Enhanced Collaboration: AI-powered platforms serve as a unified solution for finance, sales, and procurement teams, breaking down data silos and promoting transparency across departments. These tools provide all stakeholders with a single, shared source of truth for rebate performance to ensure alignment on goals and strategies. Improved visibility into rebate metrics also strengthens relationships with trading partners by enabling transparent communication and accountability, building trust across the value chain.
- Scalability: Modern AI tools are built to scale and handle increasing volumes of rebate data as businesses grow. Whether processing large-scale rebate programs, fluctuating seasonal data, or expanding operations, AI-powered platforms maintain consistent performance without bottlenecks. This scalability ensures businesses can analyze complex rebate programs and adapt to changing data volumes efficiently.
- Optimized Rebate Programs: AI facilitates real-time adjustments to rebate programs to help businesses respond dynamically to performance metrics or market conditions. By identifying trends and anomalies quickly, organizations can refine rebate terms and strategies on the fly for optimal financial outcomes. This agility improves operational responsiveness and helps businesses capitalize on opportunities while maintaining cost efficiency.
Addressing 4 Common Challenges with AI in Rebate Management
Low Data Readiness
The success of AI-driven rebate management depends heavily on clean, structured, and validated data. Without high-quality data, AI outputs will be incomplete or inaccurate, undermining decision-making and strategic outcomes. Businesses must ensure their data is accurate, consistent, and prepared for AI analysis.
The first step is data cleaning, which involves removing duplicates, correcting errors, and filling in any missing information to ensure completeness. This process eliminates inaccuracies that could distort AI-driven insights. Next, companies must focus on standardizing data formats across all systems and teams. Consistent formatting of numerical values, text, and dates ensures that AI tools can seamlessly analyze and interpret the data.
Centralizing all rebate-related data into a unified repository is equally critical. Integrating disparate systems into a single source of truth eliminates fragmentation and provides AI with a cohesive dataset to generate reliable insights. By prioritizing data readiness, businesses can lay the groundwork for AI to deliver accurate forecasts, anomaly detection, and optimized rebate strategies.
Managing Data Silos
Data silos remain a huge obstacle in rebate management, especially when information is scattered across ERP, CRM, and standalone rebate platforms. Fragmented data disrupts workflows, prevents comprehensive analysis, and limits collaboration between teams. AI-powered rebate platforms address this challenge by enabling the integration of systems to ensure a smooth flow of information across the organization.
By centralizing data from ERP, CRM, and other sources into a unified platform, businesses ensure that all stakeholders, whether in finance, sales, or procurement, have access to a single source of truth. This integration eliminates discrepancies caused by outdated or incomplete data and improves transparency. Teams can work collaboratively with up-to-date information for quicker decision-making and a more cohesive approach to rebate management.
Overcoming Planning Limitations
Traditional rebate management systems lack the agility required for dynamic financial planning and forecasting. AI addresses this limitation by delivering real-time insights that allow businesses to adjust rebate strategies quickly in response to evolving market conditions.
By analyzing historical performance data and live inputs, AI tools provide accurate, predictive forecasts that support better resource allocation and financial planning. For instance, businesses can identify underperforming rebate programs early to revise rebate terms proactively. AI also enables teams to test various scenarios to refine strategies before implementation.
This agility ensures rebate programs remain aligned with both short-term opportunities and long-term financial goals and helps businesses respond to market changes while maintaining operational efficiency.
Balancing AI and Human Expertise
AI is designed to improve, not replace, human expertise in rebate management. While AI automates repetitive processes and provides actionable insights, human oversight remains essential for critical judgment, strategic thinking, and decision-making.
AI reduces time spent on administrative busy work by handling tasks such as rebate calculations, data validation, and anomaly detection. By freeing teams from these labor-intensive activities, AI supports them in focusing on more strategic initiatives, such as analyzing performance trends, building stronger trading partner relationships, and optimizing rebate structures.
Ultimately, the role of AI is to act as a support tool that amplifies human capabilities. By combining AI’s ability to process vast datasets with human insight, businesses can achieve more informed decisions, improved rebate outcomes, and sustained commercial success.
4 Real-World Applications of AI in Rebate Management
Here are four practical examples of how AI is being applied to improve rebate management:
Predictive Forecasting for Manufacturers
AI equips manufacturers with the ability to anticipate demand shifts and adjust rebate strategies proactively. By analyzing historical sales data, market trends, and real-time inputs, AI-powered tools provide accurate demand forecasts and help manufacturers optimize rebate programs and align them with market conditions. These insights help fine-tune pricing strategies so that they remain competitive while maintaining profitability. Manufacturers can strategically adjust rebate structures to drive sales during periods of high demand or stimulate activity in underperforming segments. This data-driven approach minimizes the guesswork and supports long-term growth by keeping rebate initiatives aligned with both production capacity and market needs.
Streamlined Operations for Distributors
For distributors, managing rebate programs often involves labor-intensive tasks like manual calculations, data reconciliation, and report generation, all of which are prone to errors and delays. AI automates rebate calculations and simplifies administrative workflows to improve operational efficiency. By eliminating the need for manual intervention, AI reduces errors in rebate payouts, accelerates processing times, and ensures data accuracy across systems.
Additionally, AI tools improve procurement efficiency by providing actionable insights into supplier performance and rebate compliance. Distributors can quickly identify opportunities to optimize procurement processes, capitalize on high-performing rebate agreements, and strengthen relationships with trading partners. With AI handling routine tasks, teams can focus on strategic initiatives that drive revenue growth and improve service delivery.
Enhanced Financial Planning for Finance Leaders
AI transforms financial planning for rebate management by offering tools that detect anomalies and improve forecasting accuracy. Finance leaders can rely on AI-powered systems to identify discrepancies, such as calculation errors or outliers in rebate claims, that might otherwise go unnoticed. By addressing these issues early, businesses reduce financial risks and maintain accurate reporting.
AI also improves budget forecasting by analyzing historical rebate performance and identifying trends that inform future financial planning. Predictive modeling helps finance teams project rebate costs, assess potential financial outcomes, and align programs with overall business objectives. This clarity allows for better resource allocation and ensures rebate programs remain financially sustainable and profitable.
Improved Negotiation Leverage for Procurement Teams
AI provides procurement teams with valuable insights that strengthen negotiation leverage during vendor agreements. By analyzing rebate performance, spending patterns, and vendor trends, AI identifies areas where agreements can be optimized to align with business priorities. These insights help procurement leaders secure favorable terms while maintaining strong supplier relationships.
For example, AI tools can uncover savings opportunities by highlighting underutilized rebate programs or suggesting adjustments to deal structures. Procurement teams can use this information to negotiate rebates that drive mutual benefits, balancing supplier incentives with company profitability. By aligning vendor agreements with organizational goals, businesses achieve greater value from their rebate programs while building transparency and collaboration with trading partners.
5 Steps to Implement AI in Your Rebate Strategy
Implementing AI in your current rebate strategy can sebeem complex, but breaking it into clear, actionable steps makes the process manageable and impactful. Here are five key steps to get started:
Step 1: Assess Data Readiness
The foundation of successful AI integration lies in data readiness. Start with a data audit to identify any gaps, inconsistencies, or inaccuracies in your existing rebate data. Issues such as duplicates, missing values, or outdated information will compromise AI’s ability to deliver reliable insights. Once identified, focus on centralizing data from fragmented systems into a single, unified repository. This consolidation ensures that AI tools can access comprehensive, accurate datasets for analysis. Finally, prioritize data cleaning by correcting errors, standardizing formats, and ensuring consistency across all sources. Establishing clean, structured, and validated data sets AI up for optimal performance with actionable and trustworthy outcomes.
Step 2: Choose the Right AI-Powered Rebate Platform
Selecting the appropriate AI-powered rebate platform is crucial for maximizing value. Look for solutions that offer predictive analytics, automation capabilities, and real-time insights to address the unique challenges of rebate management. The platform should analyze vast datasets efficiently, detect anomalies, and provide actionable recommendations to optimize rebate strategies. In addition, tools with intuitive interfaces, customizable dashboards, and natural language capabilities make it easier for non-technical users to interact with AI and derive meaningful insights. A strong platform supports efficient workflows, greater accuracy, and adaptability as rebate programs become more complex.
Step 3: Start Small, Scale Gradually
Implementing AI in rebate management works best when following a crawl-walk-run strategy:
- Crawl: Begin by addressing data readiness and integrating basic AI tools for foundational processes like data validation, reporting automation, and simple analytics. At this stage, the focus is on eliminating manual inefficiencies and ensuring clean, centralized data.
- Walk: Once the basics are in place, leverage AI for targeted analytics and role-specific reporting. Use AI to identify trends, monitor performance, and provide actionable insights that improve decision-making across teams.
- Run: At the final stage, implement advanced AI features such as predictive forecasting, anomaly detection, and scenario modeling. These capabilities help businesses to optimize rebate structures proactively, identify risks, and align programs with broader financial goals. Scaling AI gradually helps organizations to adapt processes and maximize ROI while ensuring teams are ready for each phase.
Step 4: Train Teams to Work with AI
Successfully integrating AI requires that teams learn how to collaborate with the technology effectively. Start by upskilling employees to understand how AI tools work and how to extract the most value from them. Focus on teaching teams to ask the right questions and critically interpret AI-generated insights to inform strategic decisions. For example, employees should know how to identify actionable trends, validate AI findings, and apply recommendations to optimize rebate outcomes. Training employees to work alongside AI ensures that human expertise remains central to decision-making while amplifying productivity and efficiency.
Step 5: Measure and Refine
To ensure AI delivers tangible results, set clear KPIs to evaluate its impact on rebate programs. Key metrics include processing time reductions, accuracy improvements, rebate performance gains, or cost savings achieved through optimized programs. Use these benchmarks to assess the performance of AI tools and pinpoint areas for further improvement. Additionally, as AI technology evolves, continuously refine processes and explore new features that improve efficiency and performance. Regular reviews and adjustments ensure businesses stay at the forefront of innovation while maximizing the strategic value of their rebate programs.
The Future of Rebate Management is Here
AI is reshaping industries, including rebate management. By using AI-powered tools, businesses can optimize rebate processes, minimize errors, and uncover valuable insights. As AI technology advances, even more innovative solutions are likely to emerge, further transforming the future of rebate management.
As the rebate process becomes more complex, Speedy Labs ensures businesses have the tools they need to simplify rebate management.
Schedule a demo with our team to see how Speedy Labs can help you to simplify rebate management with AI.